This is because the market for finance is constantly changing and are affected by unexpected events such as economic cycles, policies changes and many other variables. Here are 10 suggestions on how to assess the model's capacity to adapt to market changes.
1. Examine Model Retraining Frequency
Why? Because the model is constantly updated to reflect the latest information and the changing market conditions.
Check that the model is able of regularly training with the latest data. Models that are retrained with updated data regularly are more apt to incorporate the most current trends and behavioral shifts.
2. Examine the effectiveness of adaptive algorithms
The reason: Certain algorithms, such as reinforcement learning as well as online models can adjust more effectively to changing patterns.
How do you determine whether the model is using adaptive algorithms that are designed to adapt to changing environments. Methods such as reinforcement learning, Bayesian networks, or the recurrent neural network with adaptable learning rates are ideal for handling shifting market dynamics.
3. Examine for the incorporation of the Regime Detection
The reason is that different market conditions (e.g. bull, bear or high volatility) can affect asset performance.
Check to see whether your model includes ways to identify regimes, such as clustering or hidden Markov Models, to be able to adjust the strategy to market conditions.
4. Examine the Sensitivity of Economic Indicators
What are the reasons Economic indicators, including the rate of interest, inflation and employment data, can have a significant impact on stock performance.
How to check whether the model includes crucial macroeconomic indicators in its inputs, allowing it to be aware of and react to economic trends that affect the market.
5. Review the model's ability to handle volatile markets
Why: Models which cannot adapt to fluctuation will fail during volatile times or cause substantial losses.
What to look for: Review past performance in volatile periods (e.g. recessions, major news events, recessions). Check for options, like dynamic adjustment of risk or volatility-targeting, which can assist models in recalibrating themselves in high-volatility periods.
6. Find out if there are any Drift detection mechanisms.
The reason: Concept drift occurs when the properties of the statistical data pertaining to the market change and affect model predictions.
How: Confirm if the model monitors for drift and adjusts its training accordingly. The use of drift-detection or changepoint detection can detect significant changes in models.
7. Check the pliability of feature engineering
What's the reason? When market conditions change, rigid feature sets may become outdated and reduce accuracy of models.
How: Look for adaptive feature engineering that allows the model to modify its features according to market trends. The dynamic selection of features or the periodic review of features can increase adaptability.
8. Evaluate Model Robustness Across Different Asset Classes
The reason is that if an algorithm is designed to work on one asset class (e.g. equities) and then it will be unable to perform well when applied to other asset types (like commodities or bonds) that behave differently.
Examine the model's flexibility by testing it on various asset classes and sectors. A model that is successful across asset classes is likely more adaptable to various market shifts.
9. Look for Ensemble or Hybrid Models to allow for flexibility
Why is that ensemble models, which combine the results of multiple algorithms, are able to overcome weaknesses and better adapt to the changing environment.
How do you determine if the model is using an ensemble approach. For example, combining mean-reversion and trend-following models. Hybrids and ensembles are able to adapt to market circumstances by switching between various strategies.
Real-time performance of major market events
Why: The test of the model's durability and adaptability against real-life events will show how robust it really is.
How: Evaluate historical performance during major disruptions in markets (e.g. COVID-19 pandemics, financial crisis). Examine the performance data that is transparent during these times to see if the model has adapted, or if the performance has decreased significantly.
By focusing on these tips and techniques, you can evaluate an AI prediction of stock prices' adaptability as it helps to ensure that it is robust and responsive amid varying market conditions. The ability to adapt will decrease risk and improve the accuracy of predictions under different economic scenarios. View the top rated artificial technology stocks for more advice including stock analysis websites, ai stock picker, ai to invest in, stock market investing, good stock analysis websites, chat gpt stock, top stock picker, ai stocks to buy now, best ai companies to invest in, chat gpt stocks and more.
Ten Best Tips On How To Evaluate The Nasdaq With An Investment Prediction Tool
In order to evaluate the Nasdaq Composite Index effectively with an AI trading predictor, it is essential to first know the distinctive aspects of the index, the technological focus and how precisely the AI is able to predict and evaluate its movement. Here are 10 top suggestions to evaluate the Nasdaq Composite using an AI Stock Trading Predictor.
1. Learn Index Composition
Why? The Nasdaq Composite includes more than 3,000 companies, mostly in the technology, biotechnology and internet industries. This makes it different from an index that is more diverse such as the DJIA.
How: Familiarize yourself with the largest and influential companies within the index, such as Apple, Microsoft, and Amazon. Knowing the impact they have on index movements can help AI models better predict overall changes.
2. Take into consideration incorporating specific sectoral factors
Why: Nasdaq stocks are significantly influenced and shaped developments in technology, news specific to the sector, and other events.
How do you include relevant variables in your AI model, for example, the performance of the tech industry, earnings reports, or trends in the software and hardware industries. Sector analysis can enhance the accuracy of the model's predictions.
3. Analysis Tools for Technical Analysis Tools
Why? Technical indicators are helpful in monitoring market sentiment and trends, especially in a highly volatile index.
How do you integrate technical analysis tools like Bollinger Bands (Moving average convergence divergence), MACD, and Moving Averages into the AI Model. These indicators can help discern buy/sell signals.
4. Monitor the impact of economic indicators on tech Stocks
Why? Economic factors, such as the rate of inflation, interest rates, and work, could affect the Nasdaq and tech stocks.
How: Incorporate macroeconomic indicators relevant for the tech sector such as trends in consumer spending as well as trends in tech investment and Federal Reserve policy. Understanding these relationships improves the model's accuracy.
5. Earnings Reports Assessment of Impact
The reason: Earnings announcements from major Nasdaq companies can cause major swings in the price and index performance.
What should you do: Make sure the model tracks earnings releases and adjusts predictions in line with these dates. Studying the price response of past earnings to earnings reports will also improve prediction accuracy.
6. Technology Stocks: Sentiment Analysis
Why: The sentiment of investors is a key element in the value of stocks. This is particularly relevant to the technology sector. Changes in trends can occur quickly.
How to incorporate sentiment analysis from social media, financial news and analyst ratings into the AI model. Sentiment metrics give context and can improve predictive capabilities.
7. Conduct backtesting with high-frequency Data
The reason: Since the Nasdaq's volatility is well known, it is important to test your forecasts using high-frequency trading.
How can you use high-frequency data for backtesting the AI model's predictions. It allows you to verify the its performance in various market conditions.
8. Evaluate the model's performance over market corrections
Why: The Nasdaq could be subject to sharp corrections. Understanding how the model works in downturns is essential.
Review the model's performance over time in the midst of major market corrections or bearmarkets. Stress testing can help reveal the model's resilience as well as its ability to limit losses in volatile times.
9. Examine Real-Time Execution Metrics
Why? Efficient execution of trades is essential to make money, particularly with a volatile index.
What are the best ways to monitor the execution metrics, such as fill rate and slippage. Examine how precisely the model can forecast optimal entry and exit times for Nasdaq related trades. This will ensure that the execution is consistent with the predictions.
10. Review Model Validation through Out-of-Sample Testing
Why? Out-of-sample tests help verify that the model generalizes well to brand new, untested data.
How to conduct rigorous tests using test-by-sample with old Nasdaq data that wasn't used to train. Compare predicted performance versus actual performance to verify that the model is accurate and reliable. model.
These guidelines will assist you to evaluate the ability of an AI stock trading prediction to accurately assess and predict changes in the Nasdaq Composite Index. Check out the recommended ai intelligence stocks for website tips including stock analysis websites, ai stock, ai companies publicly traded, stock market investing, ai to invest in, ai company stock, best ai stock to buy, new ai stocks, best sites to analyse stocks, ai stock prediction and more.
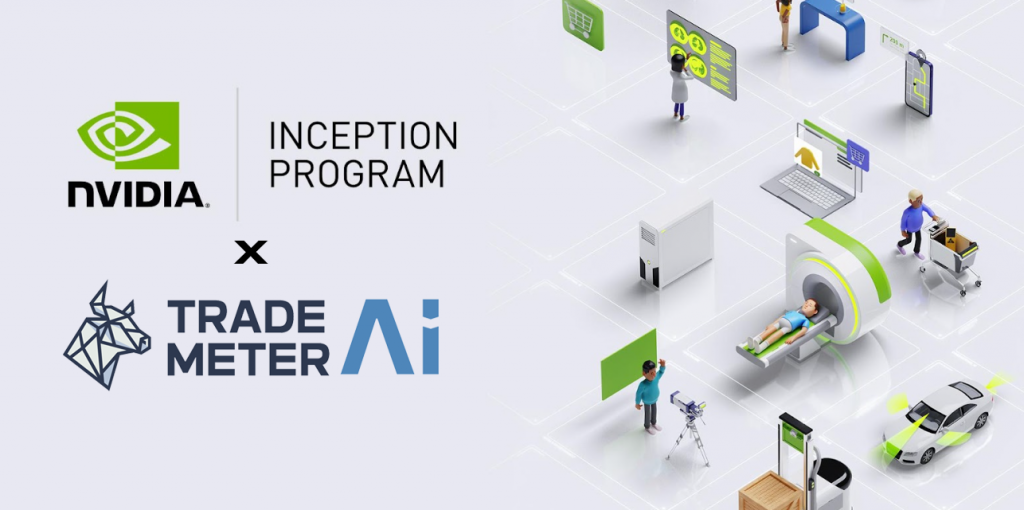